Tech companies across the U.S. are racing to develop smarter and faster AI models after China’s own Deepseek AI model shocked the world. Billed as a more energy-efficient large language model, Deepseek AI promises to reduce the power and computing costs typically associated with training and running advanced AI.
At first glance, that sounds like a win for sustainability. But there is a problem. Making AI development more accessible and less expensive doesn’t shrink demand—it fuels it. As more players enter the space and build their own models, the need for data centers to power AI will skyrocket.
Quick look:
- Deepseek AI offers energy-efficient performance lowering entry barriers for AI development.
- The model’s lean architecture enables GPT-4-level capabilities with fewer resources, attracting startups and non-tech players to build their own AI tools.
- As AI becomes more accessible, total infrastructure needs rise, fueling a surge in data center construction across the U.S.
- The “efficiency paradox” means cleaner models like Deepseek may in fact accelerate the growth of AI-powered infrastructure leading to more energy demand.
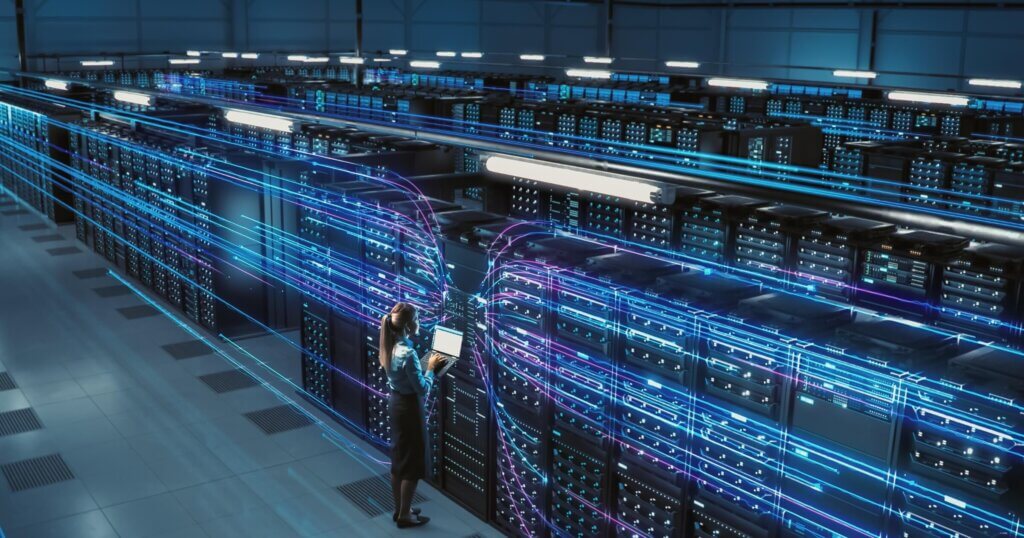
What is Deepseek AI?
Deepseek AI is a new player in the large language model space, and it’s already making waves. Developed by Chinese startup DeepSeek, the model is designed to compete with industry giants like OpenAI and Google, but with a focus on efficiency. Their flagship release, Deepseek-V2, is an open-source, general-purpose model that excels in language, reasoning, and advanced coding tasks. It’s trained on over 2 trillion tokens across multiple languages and domains, making it one of the most robust open models currently available.
At its core, Deepseek-V2 is built on transformer architecture, the same foundational tech behind models like GPT-4 and LLaMA. The company has optimized everything from training methods to token handling to reduce computational overhead while delivering impressive results across various tasks.
What does that mean in real terms?
In tests like MMLU (Massive Multitask Language Understanding) and HumanEval, Deepseek-V2 performs at a level comparable to OpenAI’s GPT-4 Turbo and Google’s Gemini 1.5, but with significantly fewer compute requirements. That balance of powerful performance with leaner processing is getting people’s attention.
In a world where computing costs and energy consumption are becoming major bottlenecks for AI, Deepseek’s efficiency-focused design is a massive step forward.
How Deepseek AI uses less power
One of the biggest selling points of Deepseek-V2 is its ability to do more with less. While many large language models chase performance at any cost, Deepseek’s approach has streamlined and optimized its architecture for efficiency, without sacrificing accuracy or speed.
So, how exactly does it use less power?
The team behind Deepseek focused heavily on token efficiency and parameter tuning. With 236 billion parameters and training done on 2 trillion tokens, Deepseek-V2 hits a sweet spot: large enough to compete with top-tier models like GPT-4, but trained in a way that minimizes redundant processing. The model supports a context length of 128K tokens and incorporates innovative architectures such as Multi-head Latent Attention (MLA) and DeepSeekMoE.
By contrast, OpenAI’s GPT-4 is a closed model with an undisclosed number of parameters, but it’s widely believed to require substantially more energy and hardware to train and operate. Even Meta’s LLaMA 2 70B model, which is often seen as a more lightweight alternative, still demands considerable infrastructure to match Deepseek’s benchmark performance.
This balance between high performance and lower compute needs is why Deepseek is being hailed as an energy-efficiency breakthrough. This will ultimately make AI architecture and models more accessible to researchers, startups, and companies without the massive cloud budgets of Big Tech.
Why data center power use is a concern
Behind every AI breakthrough is a massive data center humming with thousands of servers, pulling in power around the clock. These facilities are the backbone of AI training and deployment but are also under increasing scrutiny for how much energy they consume.
Training traditional AI models, especially large ones like GPT-4 or Gemini, can require hundreds of megawatt-hours of electricity. A single training run for a large language model can emit as much carbon as five cars over their lifetimes, and that’s just the training phase. Once a model is deployed, it still requires constant processing power to handle user queries, updates, and ongoing refinement, demanding persistent access to massive data center infrastructure.
That level of consumption has prompted environmental concerns and regulatory pressure, especially in regions with already strained energy grids. For example, 60 data centers in Virginia’s “Data Center Alley” abruptly switched to backup generators last summer, not because of a power outage but as a protective response to voltage fluctuations.
This automatic fail-safe is standard across the industry, designed to prevent damage to sensitive equipment. However, the coordinated switch-off created a massive surge of unused electricity, raising concerns among federal regulators and utility providers. With Data Center Alley consuming as much power as the entire city of Boston, events like this highlight just how critical and delicate data center energy management has become.
Much of that energy goes to keeping the hardware cool. Cooling systems are responsible for up to 40% of a data center’s total energy use, especially in older or poorly optimized facilities. Add in rising temperatures and greater server density, and it’s easy to see why location matters. Data centers are increasingly being built in cooler climates or near renewable energy sources, but that’s not always feasible, especially as demand continues to rise.
The efficiency paradox — more usage, not less
At first glance, a model like Deepseek-V2—one that delivers powerful performance with less energy—sounds like a win for sustainability. But here’s the catch: making AI development cheaper and more accessible doesn’t reduce demand. It fuels it.
This is where the efficiency paradox kicks in.
When technology becomes more efficient, the cost to use it drops. And when costs fall, more people start using it, which can lead to even higher total usage than before. This is precisely what we’re starting to see in the AI space.
With models like Deepseek, startups and smaller companies that previously couldn’t afford the sky-high computing requirements of AI training can now get into the game. That opens the door to more experimentation, custom models, and widespread AI integration across industries. From e-commerce and healthcare to education and logistics, companies that once had no shot at developing their own AI tools are now eyeing the possibilities.
This concept isn’t new. It’s known as the Jevons Paradox, first introduced in the 19th century when economist William Stanley Jevons observed that improved coal efficiency led to more coal consumption, not less. The same principle now applies to AI: the more efficient the tools become, the more widespread—and resource-hungry—their use becomes.
Data center usage has already been climbing
Even before the generative AI boom, data center usage in the U.S. had been growing steadily and rapidly. Over the past decade, the rise of cloud computing, video streaming, e-commerce, and social media has fueled massive demand for server space and processing power. With AI models becoming more prevalent, that trend is shifting into overdrive.
According to the U.S. Department of Energy, data centers already account for about 2% of total electricity use in the United States. That number is expected to grow significantly as AI adoption increases. By 2030, global data center electricity consumption could double, driven mainly by the computing demands of AI, according to a recent analysis by the International Energy Agency.
Source: International Energy Agency.
Cloud giants like Amazon Web Services, Microsoft Azure, and Google Cloud have been expanding their infrastructure at a breakneck pace to keep up with the surge in data needs. And it’s not just Big Tech. Businesses across all sectors—from finance to agriculture—increasingly rely on cloud-based services and AI tools that require robust backend infrastructure.
In the U.S., this growth has created a hotbed of data center construction, especially in regions offering tax incentives, lower land costs, and access to reliable energy. The top data center clusters include:
- Northern Virginia (Ashburn and Loudoun County): As mentioned, Data Center Alley hosts more than 275 data centers and is home to the world’s highest concentration of cloud infrastructure.
- Dallas–Fort Worth: Known for its central location and affordable power, this area has become a preferred location for large-scale data centers.
- Phoenix, Arizona: With a dry climate, cheap land, and growing solar infrastructure, Phoenix is quickly becoming a significant data center hub.
These areas are already feeling the pressure from increased demand, adding new facilities as fast as local grids and permitting systems can keep up. And with more efficient AI models lowering the barrier to entry, the demand isn’t likely to taper off anytime soon.
The future of data center construction
Even with energy-efficient models like Deepseek entering the scene, the demand for data centers shows no signs of slowing down. As AI adoption grows across industries and businesses rush to develop or deploy their own models, the infrastructure race is heating up, pushing data center construction to new heights.
One major trend shaping the future is the rise of hyperscale data centers—massive facilities designed to support thousands of servers and handle the heavy workloads of AI and cloud computing. According to Data Center Frontier, the number of hyperscale data centers globally reached 992 in 2023, with the U.S. accounting for over half of them.
We’re also seeing growth in modular and prefabricated data centers, which can be deployed faster and customized to specific use cases. These modular builds are instrumental in emerging AI markets or rural areas where traditional infrastructure might take years to establish. Some companies are even exploring AI-optimized data centers, tailored specifically for the needs of training and running large language models.
This growth comes with serious challenges. Real estate costs in popular data center hubs are climbing, power grids in high-demand areas are under pressure, and sustainability concerns are becoming harder to ignore. Balancing the need for energy with environmental responsibility is becoming a key issue, especially as more municipalities push for greener building standards and stricter emissions rules.
That’s why location matters more than ever. States like Virginia, Texas, and Arizona continue to attract data center projects due to favorable tax incentives, lower energy prices, and access to renewable power sources like solar and wind. Some companies even locate facilities near hydroelectric plants or develop partnerships with utilities to secure long-term green energy contracts.
Bottom line
Energy-efficient AI models like Deepseek significantly reduce the computational cost of building and running large language models. However, by lowering the barriers to entry, more companies—big and small—can jump into AI development, increasing overall demand for the infrastructure needed to support it. The challenge ahead is learning how to build more efficient and sustainable data centers to support this demand. Long-term success will depend on innovation, smart infrastructure planning, expanding access to clean energy, and designing more sustainable facilities to keep up with technological change.
Want more insights on the future of AI, data infrastructure, and construction trends? Subscribe to our newsletter and get timely updates delivered straight to your inbox.